The Power of Image Annotation Tools in Machine Learning
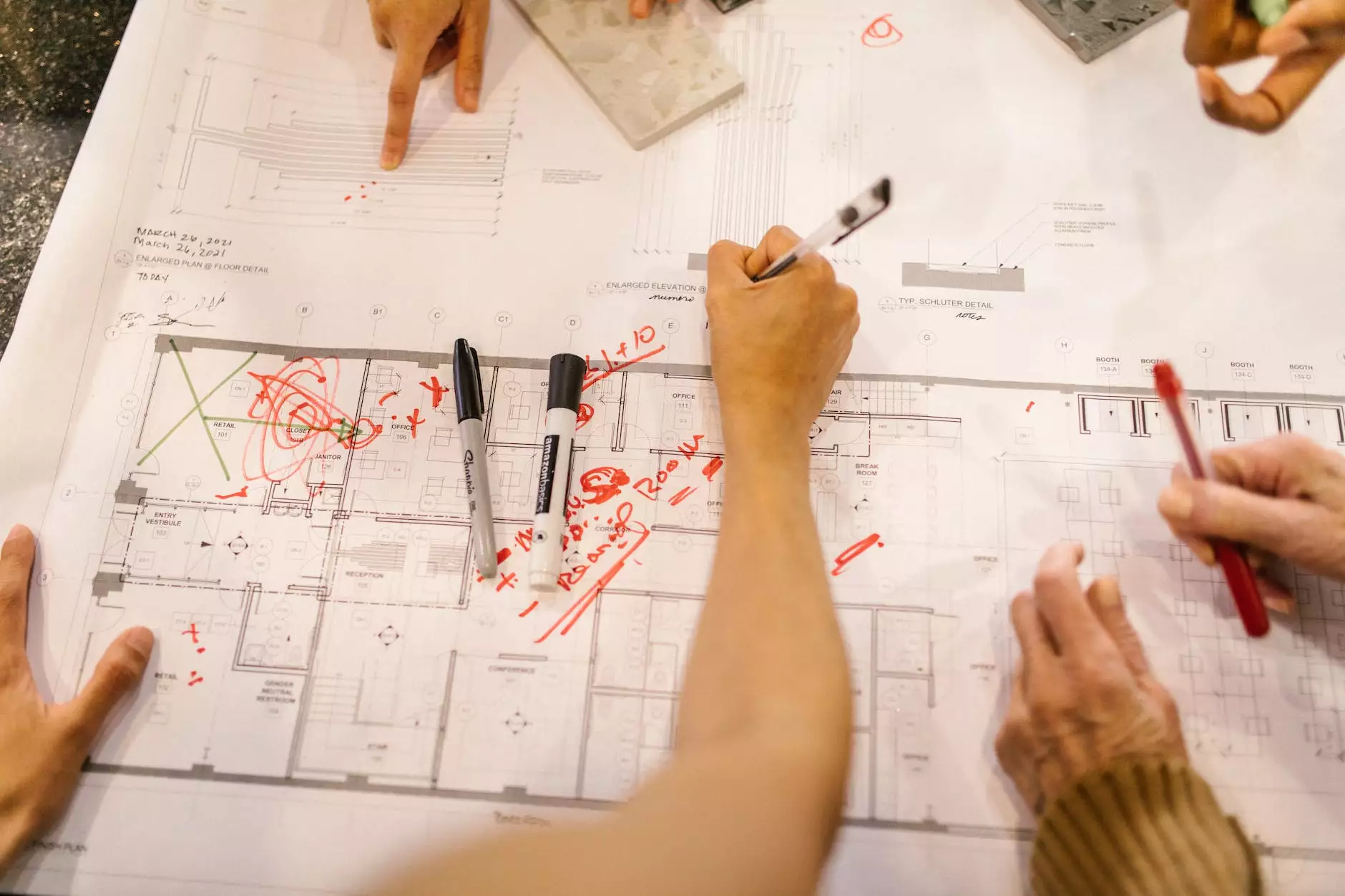
In today's fast-paced digital landscape, businesses across various sectors are embracing the power of machine learning (ML) to gain a competitive edge. One critical component of this technology is image annotation tools, which play a pivotal role in training ML models. This article will delve into the intricate details of image annotation and its broad implications for industries, including Home Services and Keys & Locksmiths. We aim to provide a comprehensive understanding that will help your business leverage these tools effectively.
Understanding Image Annotation
Image annotation refers to the process of labeling or tagging images with specific information that enables computers to understand and interpret visual data. This tagging serves as a training set for machine learning algorithms, helping them recognize patterns and make predictions. In sectors such as Home Services and locksmithing, this technology is transforming the way businesses operate.
Types of Image Annotation
- Bounding Box Annotation: Drawing boxes around objects in an image to identify and classify them.
- Semantic Segmentation: Dividing an image into regions and assigning a label to each region for a more granular understanding.
- Keypoint Annotation: Marking specific points of interest on objects, which is crucial for tasks like object detection and pose estimation.
- Polygon Annotation: Using complex shapes to annotate objects in images, allowing for accurate representation of irregularly shaped items.
The Role of Image Annotation in Machine Learning
Image annotation is not merely a trivial task; it is an essential aspect of the machine learning pipeline. It provides the necessary groundwork for model training. Without accurate annotations, the models would struggle to learn effectively, leading to poor performance in real-world applications. In the context of Home Services and locksmithing, high-quality annotations can enhance services, customer experiences, and operational efficiencies.
Improving Service Efficiency with Image Annotation Tools
In the Home Services industry, businesses often rely on visual data to assess issues or develop solutions. For instance, a plumbing company can utilize annotated images to train their ML models to identify leaks from photos submitted by customers. By applying tools for image annotation, businesses can:
- Reduce Response Times: Rapid assessment of images leads to quicker service delivery.
- Enhance Accuracy: Trained models provide precise diagnostics, leading to effective resolutions.
- Optimize Resource Allocation: Understanding prevalent issues helps in smart resource management and planning.
Streamlining the Locksmith Industry
The locksmith industry can benefit similarly from image annotation tools. Imagine a scenario where a locksmith receives images from a client stating they have lost a key. By analyzing the annotated images, ML models can identify the type of lock involved and suggest the appropriate key crafting techniques or replacement strategies. This includes:
- Quick Identification: Swiftly identifying locks and needed services based on customer-provided images.
- Better Customer Interaction: Offering informed suggestions to clients based on visual data analysis.
- Automation of Routine Tasks: Automating simple, repetitive tasks can free locksmiths to focus on more complex problems.
Choosing the Right Image Annotation Tool
With various tools available in the market, selecting the right image annotation tool is vital for optimizing your machine learning processes. Here are some factors to consider:
Key Features to Look for
- User-Friendly Interface: An intuitive interface saves valuable time and reduces training costs.
- Scalability: The tool should efficiently handle large volumes of images as your business grows.
- Collaboration Capabilities: Look for tools that allow multiple users to work simultaneously, promoting teamwork in projects.
- Integration with ML Frameworks: Ensure that the tool can seamlessly integrate with your existing machine learning platforms.
Popular Image Annotation Tools
There are several robust image annotation tools designed to cater to various business needs:
- Labelbox: A highly versatile platform that offers a collaborative approach to image annotation.
- SuperAnnotate: Focuses on seamless workflows and accurate annotation for computer vision projects.
- Scalabel: An open-source annotation tool that enables users to manage projects easily.
- VGG Image Annotator: A simple, lightweight tool ideal for educational purposes and quick projects.
Best Practices for Image Annotation
To achieve the best results from your image annotation efforts, consider the following best practices:
1. Define Clear Objectives
Before starting any annotation project, it is crucial to define clear objectives. Understanding what you want to achieve with machine learning will guide the annotation process, ensuring the data collected is relevant and useful.
2. Maintain Consistency
Consistency in labeling is vital for training effective ML models. Create guidelines for annotators to follow, ensuring all images are labeled uniformly.
3. Quality Control
Establish mechanisms for quality control to verify that annotations are accurate. This could involve periodic audits of annotated data or using automated checks to spot inconsistencies.
4. Engage Domain Experts
Involving domain experts in the annotation process can significantly improve the quality of the output. Their insights will ensure that the data reflects real-world complexities.
5. Regularly Update Datasets
As your business evolves, so should your datasets. Regularly updating annotated data is essential for maintaining the relevance and accuracy of your machine learning models.
Challenges in Image Annotation
While image annotation offers numerous benefits, several challenges need to be addressed:
1. Time Consumption
Annotating images can be a time-consuming effort. Businesses must allocate sufficient resources and time to ensure thorough and accurate annotations.
2. Subjectivity in Labeling
Different annotators may interpret and label images differently, leading to inconsistencies in the training data. Establishing clear guidelines can mitigate this issue.
3. Costs
Depending on the scale of data annotation, costs can escalate. Exploring automated annotation tools can help manage expenditures effectively.
The Future of Image Annotation in Machine Learning
The advancements in machine learning and artificial intelligence are paving the way for more sophisticated image annotation methods. Automated image annotation is emerging as a significant trend, where algorithms can label images with minimal human intervention. However, human oversight will still be crucial for validation and ensuring quality control.
Innovations to Look Out For
- Deep Learning Techniques: These can significantly reduce the time taken for annotation while improving accuracy.
- AI-Assisted Tools: Tools that employ AI to assist humans in the annotation process are set to become more prevalent.
- Cloud-Based Solutions: These allow collaboration across locations, making it easier to manage projects involving large teams.
Conclusion
The integration of image annotation tools in machine learning is not just a technological trend; it represents a transformative shift in how businesses operate. For industries such as Home Services and Keys & Locksmiths, adopting these tools can lead to streamlined processes, enhanced customer experiences, and a significant competitive advantage. By harnessing the power of image annotation, businesses can unlock new potentials and propel themselves towards a successful future in the age of digital transformation.
By understanding the nuances of image annotation and its impacts, you position your business to not only embrace current technological advancements but to thrive in an ever-evolving marketplace.
image annotation tool machine learning